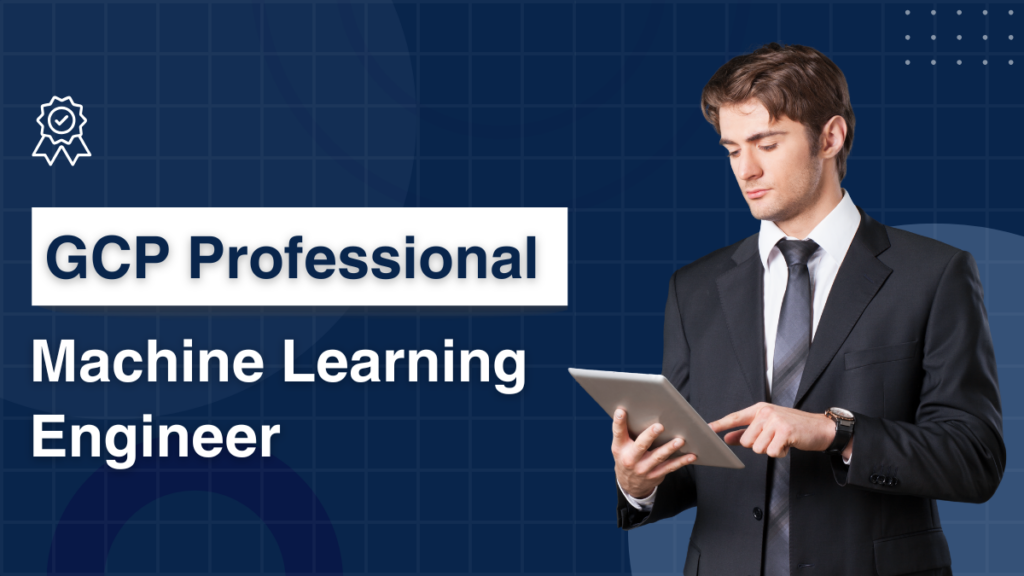
Introduction:
The GCP Professional Machine Learning Engineer certification is designed for individuals who design, build, and manage machine learning (ML) models on Google Cloud Platform (GCP). It validates expertise in implementing ML solutions that leverage GCP’s infrastructure and AI tools, focusing on automating and optimizing processes throughout the ML lifecycle.
How GCP Professional Machine Learning Engineer Helps in Career Growth
- High Demand for ML Engineers
As more industries adopt AI and machine learning technologies, the need for skilled professionals in this field has skyrocketed. With GCP certification, ML engineers are recognized for their ability to design efficient ML models and manage complex ML pipelines on a global cloud infrastructure. - Global Recognition
GCP’s ML certification is recognized worldwide, providing credibility and validation for your skills in machine learning and cloud computing. It distinguishes you as an expert capable of managing the full ML lifecycle on Google Cloud. - Broader Career Opportunities
This certification opens career pathways in fields such as AI, data science, machine learning engineering, and cloud architecture. It can lead to roles like Machine Learning Engineer, AI Solutions Architect, and Data Scientist across various industries including healthcare, finance, and technology. - Hands-On Skills in ML Pipelines and Solutions
The certification equips professionals with practical skills in designing data pipelines, developing models, and automating processes. It prepares you to handle both low-code and high-code machine learning solutions, increasing your versatility in real-world applications. - Alignment with Industry Trends
The certification ensures that you are up-to-date with the latest trends and technologies in machine learning, automation, and cloud-based AI solutions. It aligns with industry best practices for deploying and maintaining machine learning models at scale.
How to Pass the GCP Professional Machine Learning Engineer Exam
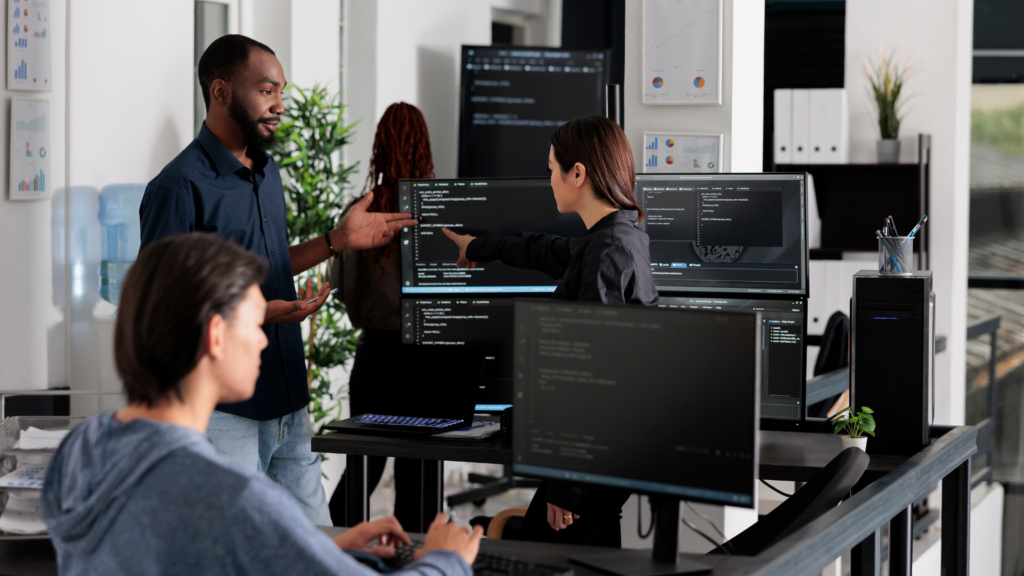
- Understand the Exam Structure
The GCP Professional Machine Learning Engineer exam consists of 60 multiple-choice questions. The exam duration is 2 hours, and the passing score is 70. The exam is categorized as intermediate in difficulty. - Key Domains to Study
The exam covers the following modules:- Architecting Low-Code ML Solutions: Learn how to design machine learning workflows using Google Cloud’s low-code and no-code tools.
- Architect Machine Learning Solutions: Focus on creating scalable, reliable machine learning systems tailored to specific business needs.
- Design Data Preparation and Processing Systems: Understand the principles of data engineering for ML, including cleaning, processing, and transformation of data.
- Develop Machine Learning Models: Master the skills to build, train, and evaluate machine learning models using GCP’s services like AI Platform and AutoML.
- Automate and Orchestrate Machine Learning Pipelines: Study the automation of ML workflows and the orchestration of data pipelines with tools like Kubeflow and Vertex AI.
- Monitor, Optimize, and Maintain Machine Learning Solutions: Learn how to continuously monitor, evaluate, and optimize models in production.
- Leverage Official Study Materials
Google Cloud offers official certification guides, hands-on labs, and practice exams. Use these resources to get a thorough understanding of GCP’s AI and ML services such as TensorFlow, BigQuery ML, and Vertex AI. - Hands-On Experience
Practical experience in developing and deploying ML models using GCP tools is crucial. Build real-world projects that involve architecting and automating ML solutions to enhance your knowledge. - Practice with Sample Questions
Use practice exams to familiarize yourself with the format and question types. This helps identify areas where you need more study and boosts your confidence going into the exam. - Engage with GCP Communities
Participating in study groups, online forums, and GCP developer communities can provide helpful insights into exam preparation strategies and real-world applications of machine learning in GCP.
Benefits of GCP Professional Machine Learning Engineer Certification
- Professional Credibility
The certification validates your ability to design and manage machine learning solutions in the cloud, demonstrating your expertise to employers and clients alike. It enhances your professional standing in the rapidly growing field of AI and ML. - Competitive Edge
GCP certification gives you a distinct advantage in the job market. With companies increasingly relying on AI and machine learning solutions, the certification sets you apart from non-certified professionals. - Increased Earning Potential
Certified GCP Machine Learning Engineers often command higher salaries due to the specialized nature of their skills. The certification enhances your earning potential and marketability. - Career Flexibility
This certification enables you to work in various industries that are adopting AI and machine learning, such as finance, healthcare, and technology. Your expertise will be applicable to a wide range of use cases, from predictive analytics to automation. - Alignment with Industry Standards
The certification ensures that you are equipped with the latest knowledge in machine learning, automation, and cloud infrastructure. It prepares you to implement solutions that align with industry best practices for ML development and deployment.
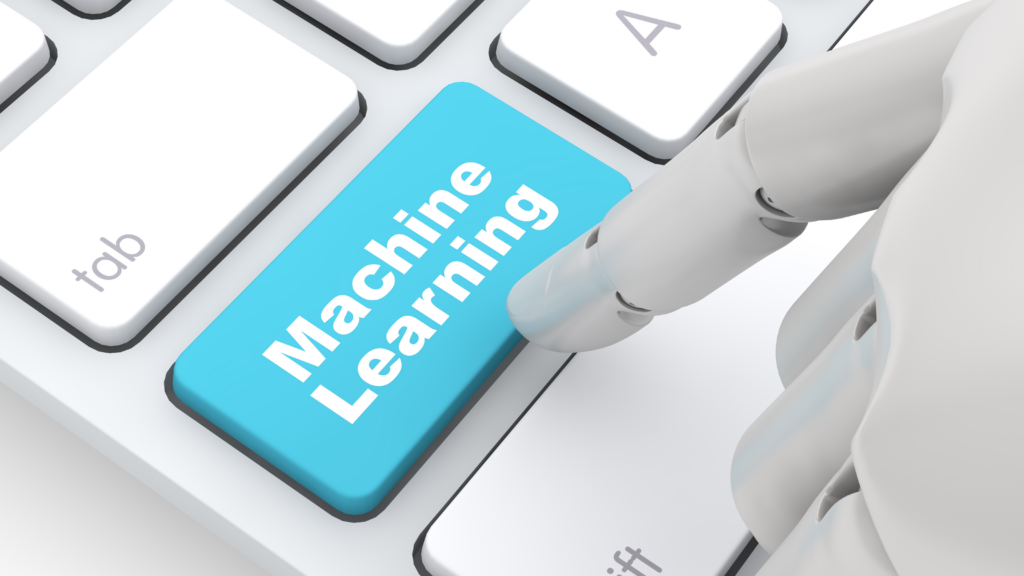
What Not to Do
- Neglecting Automation and Orchestration
A significant portion of the exam focuses on automating machine learning pipelines and orchestrating complex workflows. Overlooking this area can severely affect your performance. - Underestimating Data Preparation
Data preparation and processing are fundamental to building accurate ML models. Ignoring this module can result in a weak understanding of how to structure data for machine learning. - Focusing Only on Theory
While theoretical knowledge is essential, hands-on experience is critical for success. Make sure you have practical experience with GCP tools such as TensorFlow, BigQuery ML, and Vertex AI. - Skipping Performance Optimization
The exam tests your ability to monitor and optimize ML models in production. Failing to grasp this concept can impact your performance, as optimization is key to delivering efficient ML solutions. - Ignoring Time Management
With 60 questions to answer in just 2 hours, effective time management is crucial. Ensure you pace yourself properly during the exam to complete all questions.